Fine-tuning a Transformer for text classification
I have found myself often enough trying to classify a text that I decided to be lazy and write a solution that I could always rely on.
The following script is an example on how to pre-train a HuggingFace transformer for text classification. This example uses only two classes, identifying whether the sentiment of a text is positive or negative, but nothing stops you from adding more classes as long as you predict just one.
There are no difficult imports that I can tell - I think you can install all the required libraries with the command
pip install transformers datasets evaluate nltk
but I'll double check just in case.
Update: I have changed the script to extract the function get_dataset_from_json_files()
.
You can use this function as is to replace the calls to get_data()
. I also updated the prediction code to give an example of
how to make predictions for a lot of texts at once.
import json
import numpy as np
import os
import random
import tempfile
# These are all HuggingFace libraries
from datasets import load_dataset
import evaluate
from transformers import DistilBertTokenizer, DistilBertForSequenceClassification,\
TrainingArguments, Trainer, TextClassificationPipeline
# KeyDataset is a util that will just output the item we're interested in.
from transformers.pipelines.pt_utils import KeyDataset
# Library for importing a sentiment classification dataset.
# Only here for demo purposes, as you would use your own dataset.
from nltk.corpus import movie_reviews
# The BERT tokenizer is always the same so we declare it here globally.
tokenizer = DistilBertTokenizer.from_pretrained("distilbert-base-uncased")
def get_data():
""" Returns a dataset built from a toy corpora.
Returns
-------
Dataset
An object containing all of our training, validation, and test data.
This object stores all the information about a text, namely, the raw
text (which is stored both as text and as numeric tokens) and the
proper class for every text.
Notes
-----
If you have texts in this format already then you can skip most of this
function and simply jump to the `get_dataset_from_files` function.
References
----------
Most of this function is taken from the code in
https://huggingface.co/course/chapter5/5?fw=pt.
Note that that link is very confusing at times, so if you read that code
remember to be patient.
"""
# We read all of our (toy) data from NLTK. The exception catches the case
# where we haven't downloaded it yet and downloads it.
try:
all_ids = movie_reviews.fileids()
except LookupError:
import nltk
nltk.download('movie_reviews')
all_ids = movie_reviews.fileids()
# We identify texts by their ID. Given that we have those IDs now, we
# proceed to shuffle them and assign each one to a specific split.
# Note that we fix the random seed to ensure that the datasets are always
# the same across runs. This is not strictly necessary after creating a
# dataset object (because we want to reuse it), but it is useful if you
# delete that dataset and want to recreate it from scratch.
random.seed(42)
random.shuffle(all_ids)
num_records = len(all_ids)
# This dictionary holds the name of the temporay files we will create.
tmp_files = dict()
# We assigne the IDs we read before to their data splits.
# Note that we use a fixed 80/10/10 fixed split.
for split in ['train', 'val', 'test']:
if split == 'train':
split_ids = all_ids[:int(0.8 * num_records)]
elif split == 'val':
split_ids = all_ids[int(0.8 * num_records):int(0.9 * num_records)]
else:
split_ids = all_ids[int(0.9 * num_records):]
all_data = []
# We read the data one record at the time and store it as dictionaries.
for text_id in split_ids:
# We read the text.
text = movie_reviews.raw(text_id)
# We read the class and store it as an integer.
if movie_reviews.categories(text_id)[0] == 'neg':
label = 0
else:
label = 1
all_data.append({'id': text_id, 'text': text, 'label': label})
# Step two is to save this data as a list of JSON records in a temporary
# file. If you use your own data you can simply generate these files as
# JSON from scratch and save yourself this step.
# Note that we also save the name of the temporary file in a dictionary
# so we can re-read it later.
tmp_file = tempfile.NamedTemporaryFile(delete=False)
tmp_files[split] = tmp_file.name
tmp_file.write(json.dumps(all_data).encode())
tmp_file.close()
# Step three is to create a data loader that will read the data we just
# saved to disk.
dataset = get_dataset_from_json_files(tmp_files['train'], tmp_files['val'], tmp_files['test'])
# Remove the temporary files and return the tokenized dataset.
for _, filename in tmp_files.items():
os.unlink(filename)
return dataset
def get_dataset_from_json_files(train_file, val_file=None, test_file=None):
""" Given a set of properly-formatted files, it reads them and returns
a Dataset object containing all of them.
Parameters
----------
train_file : str
Path to a file containing training data.
val_file : str
Path to a file containing validation data.
test_file : str
Path to a file containing test data.
Returns
-------
Dataset
An object containing all of our training, validation, and test data.
This object stores all the information about a text, namely, the raw
text (which is stored both as text and as numeric tokens) and the
proper class for every text.
Notes
-----
IMPORTANT: the first time you run this code, the resulting dataset is saved
to a temporary file. The console will tell you where it is (in Linux it is
/home/<user>/.cache/huggingface/datasets/...). This temporary location is
used in all subsequent calls, so if you change your dataset remember to
remove this cached file first!
This function is a thin wrapper around the `load_dataset` function where
we hard-coded the file format to use JSON.
If you don't want to read that function's documentation, it is enough to
provide files whose content is simply a JSON list of dictionaries, like so:
[{'id': '1234', 'text': 'My first text', 'label': 0}, {'id': '5678', 'text': 'My second text', 'label': 1}]
"""
files_dict = {'train': train_file}
if val_file is not None:
files_dict['val'] = val_file
if test_file is not None:
files_dict['test'] = test_file
dataset = load_dataset('json', data_files=files_dict)
# Tokenize the data
def tokenize_function(examples):
return tokenizer(examples['text'], padding='max_length', truncation=True)
tokenized_dataset = dataset.map(tokenize_function, batched=True)
# Return the tokenized dataset.
return tokenized_dataset
if __name__ == '__main__':
# In which mode we want to use this script
# 'train': start from a pre-trained model, fine-tune it to a dataset, and then
# save the resulting model
# 'test': evaluate the performance of a fine-tuned model over the test data.
# 'predict': make predictions for unseen texts.
mode = 'predict'
assert mode in ['train', 'test', 'predict'], f'Invalid mode {mode}'
# We define here the batch size and training epochs we want to use.
batch_size = 16
epochs = 10
# Where to save the trained model.
trained_model_dir = 'training_output_dir'
if mode == 'train':
# Train mode. Code adapted mostly from:
# https://huggingface.co/docs/transformers/training#train-with-pytorch-trainer.
# Collect the training data
inputs = get_data()
# Since we are starting from scratch we download a pre-trained bert model
# from HuggingFace. Note that we are hard-coding the number of classes here!
model = DistilBertForSequenceClassification.from_pretrained("distilbert-base-uncased", num_labels=2)
# Define training arguments
# You can define a loooot more hyperparameters - see them all in
# https://huggingface.co/docs/transformers/v4.26.1/en/main_classes/trainer#transformers.TrainingArguments
training_args = TrainingArguments(output_dir=trained_model_dir,
evaluation_strategy='epoch',
per_device_train_batch_size=batch_size,
num_train_epochs=epochs,
learning_rate=1e-5)
# Define training evaluation metrics
metric = evaluate.load('accuracy')
def compute_metrics(eval_pred):
logits, labels = eval_pred
predictions = np.argmax(logits, axis=-1)
return metric.compute(predictions=predictions, references=labels)
# Define the trainer object and start training.
# The model will be saved automatically every 500 epochs.
trainer = Trainer(model=model,
args=training_args,
train_dataset=inputs['train'],
eval_dataset=inputs['val'],
compute_metrics=compute_metrics)
trainer.train()
elif mode == 'test':
# Perform a whole run over the validation set.
# This code is adapted mostly from:
# https://huggingface.co/docs/evaluate/base_evaluator
# Some useful information also here:
# https://huggingface.co/docs/datasets/metrics
# Collect the validation data
inputs = get_data()
# Create our evaluator
task_evaluator = evaluate.evaluator("text-classification")
# Use the model we trained before to predict over the validation data.
# Note that we are providing the name of the classes by hand. We are not
# using these labels here, but it is useful for the prediction branch.
model = DistilBertForSequenceClassification.from_pretrained(f'./{trained_model_dir}/checkpoint-1000/',
num_labels=2,
id2label={0: 'negative', 1: 'positive'})
# Define the evaluation parameters. We use the common text evaluation
# measures, but there are plenty more.
eval_results = task_evaluator.compute(
model_or_pipeline=model,
tokenizer=tokenizer,
data=inputs['test'],
metric=evaluate.combine(['accuracy', 'precision', 'recall', 'f1']),
label_mapping={"negative": 0, "positive": 1}
)
# `eval_results` is a dictionary with the same keys we defined in
# the `metric` parameters, plus some time measures.
print(eval_results)
elif mode == 'predict':
# Make predictions for individual texts.
# Same as above, we use the model we trained before to predict a single text.
model = DistilBertForSequenceClassification.from_pretrained(f'./{trained_model_dir}/checkpoint-1000/',
num_labels=2,
id2label={0: 'negative', 1: 'positive'})
# We build a text classification pipeline.
# Note that `top_k=None` gives us probabilities for every class while
# `top_k=1` returns values for the best class only.
# Inspired on https://discuss.huggingface.co/t/i-have-trained-my-classifier-now-how-do-i-do-predictions/3625
pipe = TextClassificationPipeline(model=model, tokenizer=tokenizer,
batch_size=batch_size, top_k=None)
sequences = ['Hello, my dog is cute', 'Hello, my dog is not cute']
predictions = pipe(sequences)
print(predictions)
# The above print shows something like:
# [[{'label': 'positive', 'score': 0.574},
# {'label': 'negative', 'score': 0.426}],
# [{'label': 'negative', 'score': 0.868},
# {'label': 'positive', 'score': 0.132}]]
# For a more heavy-duty approach we now classify an entire dataset.
inputs = get_data()
test_inputs = inputs['test']
outputs = []
# Note that 'text' is the key we defined in the `get_data` function.
for out in pipe(KeyDataset(test_inputs, 'text'), batch_size=batch_size, truncation=True, max_length=512):
outputs.append(out)
# Now that we collected all outputs we massage them a little bit for
# a more friendly format. For every prediction we will print a line like:
# 'pos/cv093_13951.txt: positive (0.996)'
for i in range(len(test_inputs)):
id = test_inputs[i]['id']
pred_label = outputs[i][0]['label']
pred_prob = outputs[i][0]['score']
print(f'{id}: {pred_label} ({pred_prob})')
Thoughts on ChatGPT, LLMs, and search engines
Now that everyone is talking about Large Language Models (LLMs) in general and ChatGPT in particular I thought I would share a couple thoughts I've been having about this technology that I haven't seen anywhere else. But first, let's talk about chess.
Chess is a discipline notorious for coming out on top when the robots came to take its lunch. Once it became clear that even the humblest of PCs can play better than a World Chess Champion, the chess community started using these chess engines to improve their games and learn new tactics. Having adopted these engines as a fact of life, chess is as popular as ever (if not more). Sure, a computer can do a "better" job on a fraction of the time, but who cares? It's not like there's an unmet economic need for industrial-strength chess players.
In contrast, one field that isn't doing as well is digital art. With the advent of diffusion models like Midjourney and Stable Diffusion many artists are worried that their livelihoods are now at stake and are letting the world know, ranging from comics (I like this one more but this one hits closer to home) all the way to class action lawsuits. My quick take is that these models are here to stay and that they won't necessarily destroy art but they will probably cripple the art business the same way cheap restaurants download food pictures from the internet instead of paying a professional photographer.
LLMs are unusual in that sense because they aren't disrupting a field as much as a means of communication. "Chess player" is something one does, and so is "artist"1. But "individual who uses language" is on a different class altogether, and while some of the effects of these technologies are easy to guess, others not so much.
Random predictions
What are we likely to see in the near future? The easiest prediction is a rise in plagiarism. Students are already using ChatGPT to generate essays regardless of whether the output makes sense or not. And spam will follow closely behind: we are already awash in repost spam and ramblings disguised as recipes, but once people start submitting auto-generated sci-fi stories to magazines we will see garbage in any medium that offers even the slightest of rewards, financial or not. Do you want a high-karma account on Reddit to establish yourself as not-a-spammer and use it to push products? Just put your payment information here and the robots will comment for you. No human interaction needed2.
What I find more interesting and likely more disruptive is the replacement of search engines with LLMs. If I ask ChatGPT right now about my PhD advisor I get an answer - this particular answer happens to be all wrong3, but let's pretend that it's not 4. This information came from some website, but the system is not telling me which one. And here there's both an opportunity and a risk. The opportunity is the chance of cutting through the spam: if I ask for a recipe for poached eggs and I get a recipe for poached eggs then I no longer have to waddle through long-winded essays on how poached eggs remind someone of evening at their grandma's house. On the other hand, this also means that all the information we collectively placed on the internet would be used for the profit of some company without even the meagre attributions we currently get.
On the long tail, and entering into guessing territory, it would be tragic if people started writing like ChatGPT. These systems have a particular writing style composed of multiple short sentences and it's not hard to imagine that young, impressionable people may start copying this output once it is widespread enough. This has happened before with SMS, so I don't see why it couldn't happen again.
Pointless letters and moving forward
One positive way to move forward would be to accept that a lot of our daily communication is so devoid of content that even a computer with no understanding of the real world can do it and work on that.
When I left my last job I auto-generated a goodbye e-mail with GPT-3, and the result was so incredibly generic that no one would have been able to learn anything from it. On the other hand, I doubt anyone would have noticed: once you've read a hundred references to "the good memories" you no longer stop to wonder whether there were any good memories to begin with. I didn't send that auto-generated e-mail. In fact, I didn't send anything: I had already said goodbye in person to the people that knew me and there was no reason to say anything else. The amount of information that was conveyed was exactly the same, but my solution wasted less of other people's time.
Maybe this is our opportunity to freshen up our writing and start writing interestingly, both in form (long sentences for the win!) and in content. The most straightforward solution would be cursing: these models have to be attractive to would-be investors they are strictly programmed not to use curse words and NSFW content (I just tried). So there's a style that no AI will be copying in the near future.
Footnotes
- Note that this is a simplification for the sake of the argument. As someone who often said "being a programmer is both what I do and what I am" I am aware that "artist" (like so many other professions) isn't just a job but also a way of looking at the world.
- I have noticed that people on Reddit will upvote anything without reading it first, so this is not a high bar to clear.
- The answer mashed together several researchers into one. One could argue that I got more researchers per researcher, which is definitely a take.
- The answer doesn't have to be correct - all it takes is for the person using the system to believe that the answer is correct, something we are already seeing despite the overwhelming evidence to the contrary.
GPT-3 is blockchain
I need to share with you an epiphany that occurred to me yesterday.
Have you heard of GPT-3? If not, I can tell you that it's a language model that has been showing up everywhere. Having been trained with a lot of data, it can generate text that people find impressive.
If you follow the hype, GPT-3 will revolutionize everything - people have been using it to generate plausible-looking creative fiction, pickup lines, SQL Queries that are sometimes wrong, trivia answers, tweets, and so on.
But you know what no one has generated yet, as far as I know? Something useful. Or even better: something that people always wanted but current technology cannot provide.
People are excited about GPT-3 because it promises to "just work" - you give it the right prompt and you get the right answer. This would solve all of those pesky problems associated with NLP such as "this search terms make no sense", "I hate knowledge bases", "That question has multiple answers", or "I don't want to manually write all possible answers for my system". But this is not what GPT-3 can do, because GPT-3 will not bend to your so-called facts and therefore will not do what you want. As Robert Dale puts it when talking about GPT-2: "driven as it is by information that is ultimately about language use, rather than directly about the real world, it roams untethered to the truth". In other words, people are excited about GPT-3 because they think it solves a different problem that the one it actually does.
If you want a chatbot to tell a patient that the solution to their depression is talking to a professional instead of GPT-3's suggestion that they should just go ahead and kill themselves, you need a way to constrain the system's output. This means that you still need to write the code that interprets the patient's problems, the code that chooses the right solution to that problem, and the code that says exactly what you want, no more and no less. And while turning structured data into human-readable sentences is a valid possible use for GPT-3, the amount of work required to constrain its output to an acceptable error level is comparable to the effort required to write smart templates that guarantee you'll generate exactly what you want.
And so, GPT-3 joins blockchain technology in being a solution searching for a problem. In fact, the parallels are kind of amazing: both technologies are hyped to the extreme, completely misunderstood by the general public, very expensive to run, and products based on them rarely make it out of the proof-of-concept stage.
I would like to leave you with two optimistic thoughts. First, I do think that it is only a matter of time before someone actually finds a good use for GPT-3. I predict it is going to be something marginal, with my best bet being something related to grammatical correctness. Abstractive summarization is also a good candidate, but my faith is lower because inserting unrelated facts is simultaneously what abstractive summarization tries to avoid and what GPT-3 does best.
And second, I want to let you know that there's a great business opportunity here. The blockchain craze reached the point where simply putting "blockchain" in your company name is enough to make your stock price rise by 289 percent. Therefore, if my prediction is correct then all you need to do is either name your own company "GPT-3" or invest in someone else doing it. Sure, their stock will probably tank once investors realize they invested for the wrong reasons, but by then you will have hopefully cashed out and moved on to something else.
Disclaimer: I am not an investment banker, this post does not constitute financial advice, I don't know why anyone would listen to me, and you shouldn't follow advice you find on random blog posts anyway.
A more polite Taylor Swift with NLP and word vectors
My relation with Taylor Swift is complicated: I don't hate her — in fact, she seems like a very nice person. But I definitely hate her songs: her public persona always comes up to me as entitled, abusive, and/or an unpleasant person overall. But what if she didn't have to be? What if we could take her songs and make them more polite? What would that be like?
In today's post we will use the power of science to answer this question. In particular, the power of Natural Language Processing (NLP) and word embeddings.
The first step is deciding on a way to model songs. We will reach into our
NLP toolbox and take out
Distributional
semantics, a research area that
investigates whether words that show up in similar contexts also have similar
meanings. This research introduced the idea that once you treat a word like a
number (a vector, to be precise, called the embedding of the word), you
can apply regular math operations to it and obtain results that make sense.
The classical example is a result shown in
this paper, where
Mikolov and his team managed to represent words in such a way that the
result of the operation King - man + woman
ended up being very
close to Queen
.
The picture below shows an example. If we apply this technique to all the Sherlock Holmes novels, we can see that the names of the main characters are placed in a way that intuitively makes sense if you also plot the locations for "good", "neutral", and "evil" as I've done. Mycroft, Sherlock Holmes' brother, barely cares about anything and therefore is neutral; Sherlock, on the other hand, is much "gooder" than his brother. Watson and his wife Mary are the least morally-corrupt characters, while the criminals end up together in their own corner. "Holmes" is an interesting case: the few sentences where people refer to the detective by saying just "Sherlock" are friendly scenes, while the scenes where they call him "Mr. Holmes" are usually tense, serious, or may even refer to his brother. As a result, the world "Sherlock" ends up with a positive connotation that "Holmes" doesn't have.
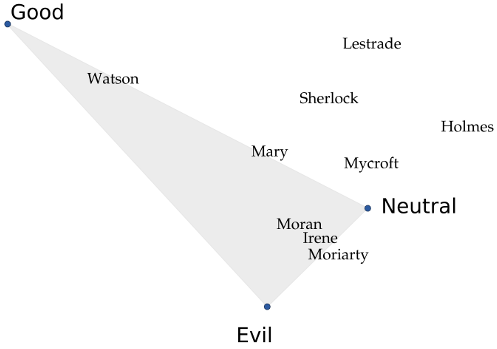
This technique is implemented by
word2vec, a series of
models that receive documents as input and turn their words into vectors.
For this project, I've chosen the
gensim Python library. This
library does not only implement word2vec
but also
doc2vec
, a model that will do all the heavy-lifting for us when it
comes to turn a list of words into a song.
This model needs data to be trained, and here our choices are a bit limited. The biggest corpus of publicly available lyrics right now is (probably) the musiXmatch Dataset, a dataset containing information for 327K+ songs. Unfortunately, and thanks to copyright laws, working with this dataset is complicated. Therefore, our next best bet is this corpus of 55K+ songs in English, which is much easier to get and work with.
The next steps are more or less standard: for each song we take their words,
convert them into vectors, and define a "song" as a special word whose meaning
is a combination of its individual words. But once we have that, we can start
performing some tests. The following code does all of this, and then asks an
important question: what would happen if we took Aerosmith's song
Amazing,
removed the
import csv
import gzip
from gensim.models import Doc2Vec
from gensim.models.doc2vec import TaggedDocument
documents = []
with gzip.open('songlyrics.zip', 'r') as f:
csv_reader = csv.DictReader(f)
counter = 0
# Read the lyrics, turn them into documents,
# and pre-process the words
for row in csv_reader:
words = simple_preprocess(row['text'])
doc = TaggedDocument(words, ['SONG_{}'.format(counter)})
documents.append(doc)
counter += 1
# Train a Doc2Vec model
model = Doc2Vec(documents, size=150, window=10, min_count=2, workers=10)
model.train(document, total_examples=len(documents), epochs=10)
# Apply some simple math to a song, and obtain a list of the 10
# most similar songs to the result.
# In our lyrics database, song 22993 is "Amazing", by Aerosmith
song = model['SONG_22993']
query_vector = song - model['amazing']
for song, vector in model.docvecs.most_similar([query_vector]):
print(song)
One would expect that
- ...Margarita, a song about a man who meets a woman in a bar and cooks soup with her.
- ...Alligator, a song about an alligator lying by the river.
- ...Pony Express, a song about a mailman delivering mail.
We can use this same model to answer all kind of important questions I didn't know I had:
- Have you ever wondered what would be "amazingly lame"? I can tell you!
Amazing +lame = History in the making, a song where a rapper tells us how much money he has. - Don't you think sometimes "I like
We
are the World, but I wish it had more
violence ?". If so, Blood on the World's hands is the song for you. - What if we take Roxette's
You
don't understand me and add
understanding to it? As it turns out, we end up with It's you, a song where a man breaks up with his wife/girlfriend because he can't be the man she's looking for. I guess he does understand her now but still: dude, harsh. - On the topic of hypotheticals: if we take John Lennon's
Imagine
and we take away the
imagination , all that's left is George Gershwin's Strike up the band, a song about nothing but having "fun, fun, fun". On the other hand, if we added even moreimagination we end up with Just my imagination, dreaming all day of a person who doesn't even know us.
This is all very nice, but what about our original question: what if we took Taylor Swift's songs and removed all the meanness? We can start with her Grammy-winning songs, and the results are actually amazing: the song that best captures the essence of Mean minus the meanness is Blues is my middle name, going from a song where a woman swears vengeance to a song where a man quietly laments his life and hopes that one day things will come his way. Adding politeness to We are never coming back together results in Everybody knows, a song where a man lets a woman know he's breaking up with her in a very calm and poetic way. The change is even more apparent when the bitter Christmases when you were mine turns into the (slightly too) sweet memories of Christmas brought by Something about December.
Finally, and on the other side, White Horse works better with the anger in. While this song is about a woman enraged at a man who let her down, taking the meanness out results in the hopeless laments of Yesterday's Hymn.
So there you have it. I hope it's clear that these are completely accurate results, that everything I've done here is perfectly scientific, and that any kind of criticism from Ms. Swift's fans can be safely disregarded. But on a more serious note: I hope it's clear that this is only the tip of the iceberg, and that you can take the ideas I've presented here in many cool directions. Need a hand? Let me know!
Further reading
- Piotr Migdal has written a
popular
post about why the
King - man + woman = Queen
analogy works, including an interactive tool. - The base for my code was inspired by
this
tutorial on the use of
word2vec
. - The good fellows at FiveThirtyEight used this technique to analyze what Trump supporters look like, applying the technique to the news aggregator Reddit.
The Tapiz instruction-giving system
This article is the third of a series in which I explain what my research is about in (I hope) a simple and straightforward manner. For more details, feel free to check the Research section.
For my first research paper during my PhD, the basic idea was pretty simple. Imagine that, after recording several hours of people being guided around a room, I realize the following: everytime a player stood in front of a door, and someone told them "go straight", they walked through the door. So now I ask: if you are standing in front of a door, and I want you to walk through it, would it be enough for me to say "go straight", like before? My research team and I wanted to give this question an answer, so this is what we did.
We looked at our recorded data. Whenever we saw a player moving somewhere, we took notes about where the player was, where is the player now, and what was the instruction that convinced the player to move from one place to the other. We then created a big dictionary, where each entry reads "to move the player from point A to point B, say this". Quite smart, right?
The most important part about this idea is that we don't need to teach our computer how to understand language - in fact, when our system reads "turn right" in our dictionary, it has no idea about what "turn" or "right" mean. All our system cares about is that saying "turn right", for some strange reason, causes people to look to the right. This makes our system a lot simpler than other systems that try to understand everything.
Now, let's complicate things a bit: let's say I tell you "walk through the door to your left". You turn left, walk through the door, take 7 steps, give a full turn to look at the room, and then you wait for me to say something else. Which of those things you did because I told you, and which ones you did because you felt like it?
Since we didn't really know the answer, we tried two ideas: in the first case, we decided that everything you did was a reaction to our instruction (including the final turn), while in the second one we only considered the first action (turning left), and nothing else. As you can see, neither approach is truly correct: one is too short, and the other one is too long. But in research we like trying simple ideas first, and we decided to give these two a try.
Our results showed that the second approach works better, because if you advance just one step I can guide you to the next, but if you do too many things at once there's a chance you'll get confused and lost. Also, since our system is repeating what other humans said before, players thought the instructions were not too artificial.
Not bad for my first project, right?