A more polite Taylor Swift with NLP and word vectors
My relation with Taylor Swift is complicated: I don't hate her — in fact, she seems like a very nice person. But I definitely hate her songs: her public persona always comes up to me as entitled, abusive, and/or an unpleasant person overall. But what if she didn't have to be? What if we could take her songs and make them more polite? What would that be like?
In today's post we will use the power of science to answer this question. In particular, the power of Natural Language Processing (NLP) and word embeddings.
The first step is deciding on a way to model songs. We will reach into our
NLP toolbox and take out
Distributional
semantics, a research area that
investigates whether words that show up in similar contexts also have similar
meanings. This research introduced the idea that once you treat a word like a
number (a vector, to be precise, called the embedding of the word), you
can apply regular math operations to it and obtain results that make sense.
The classical example is a result shown in
this paper, where
Mikolov and his team managed to represent words in such a way that the
result of the operation King - man + woman
ended up being very
close to Queen
.
The picture below shows an example. If we apply this technique to all the Sherlock Holmes novels, we can see that the names of the main characters are placed in a way that intuitively makes sense if you also plot the locations for "good", "neutral", and "evil" as I've done. Mycroft, Sherlock Holmes' brother, barely cares about anything and therefore is neutral; Sherlock, on the other hand, is much "gooder" than his brother. Watson and his wife Mary are the least morally-corrupt characters, while the criminals end up together in their own corner. "Holmes" is an interesting case: the few sentences where people refer to the detective by saying just "Sherlock" are friendly scenes, while the scenes where they call him "Mr. Holmes" are usually tense, serious, or may even refer to his brother. As a result, the world "Sherlock" ends up with a positive connotation that "Holmes" doesn't have.
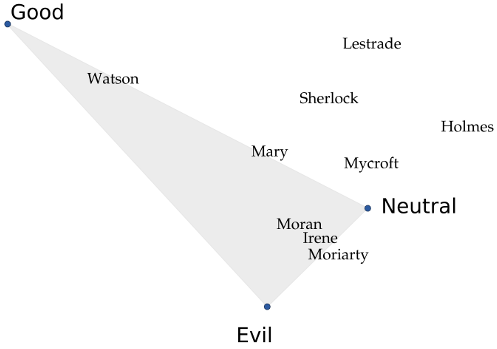
This technique is implemented by
word2vec, a series of
models that receive documents as input and turn their words into vectors.
For this project, I've chosen the
gensim Python library. This
library does not only implement word2vec
but also
doc2vec
, a model that will do all the heavy-lifting for us when it
comes to turn a list of words into a song.
This model needs data to be trained, and here our choices are a bit limited. The biggest corpus of publicly available lyrics right now is (probably) the musiXmatch Dataset, a dataset containing information for 327K+ songs. Unfortunately, and thanks to copyright laws, working with this dataset is complicated. Therefore, our next best bet is this corpus of 55K+ songs in English, which is much easier to get and work with.
The next steps are more or less standard: for each song we take their words,
convert them into vectors, and define a "song" as a special word whose meaning
is a combination of its individual words. But once we have that, we can start
performing some tests. The following code does all of this, and then asks an
important question: what would happen if we took Aerosmith's song
Amazing,
removed the
import csv
import gzip
from gensim.models import Doc2Vec
from gensim.models.doc2vec import TaggedDocument
documents = []
with gzip.open('songlyrics.zip', 'r') as f:
csv_reader = csv.DictReader(f)
counter = 0
# Read the lyrics, turn them into documents,
# and pre-process the words
for row in csv_reader:
words = simple_preprocess(row['text'])
doc = TaggedDocument(words, ['SONG_{}'.format(counter)})
documents.append(doc)
counter += 1
# Train a Doc2Vec model
model = Doc2Vec(documents, size=150, window=10, min_count=2, workers=10)
model.train(document, total_examples=len(documents), epochs=10)
# Apply some simple math to a song, and obtain a list of the 10
# most similar songs to the result.
# In our lyrics database, song 22993 is "Amazing", by Aerosmith
song = model['SONG_22993']
query_vector = song - model['amazing']
for song, vector in model.docvecs.most_similar([query_vector]):
print(song)
One would expect that
- ...Margarita, a song about a man who meets a woman in a bar and cooks soup with her.
- ...Alligator, a song about an alligator lying by the river.
- ...Pony Express, a song about a mailman delivering mail.
We can use this same model to answer all kind of important questions I didn't know I had:
- Have you ever wondered what would be "amazingly lame"? I can tell you!
Amazing +lame = History in the making, a song where a rapper tells us how much money he has. - Don't you think sometimes "I like
We
are the World, but I wish it had more
violence ?". If so, Blood on the World's hands is the song for you. - What if we take Roxette's
You
don't understand me and add
understanding to it? As it turns out, we end up with It's you, a song where a man breaks up with his wife/girlfriend because he can't be the man she's looking for. I guess he does understand her now but still: dude, harsh. - On the topic of hypotheticals: if we take John Lennon's
Imagine
and we take away the
imagination , all that's left is George Gershwin's Strike up the band, a song about nothing but having "fun, fun, fun". On the other hand, if we added even moreimagination we end up with Just my imagination, dreaming all day of a person who doesn't even know us.
This is all very nice, but what about our original question: what if we took Taylor Swift's songs and removed all the meanness? We can start with her Grammy-winning songs, and the results are actually amazing: the song that best captures the essence of Mean minus the meanness is Blues is my middle name, going from a song where a woman swears vengeance to a song where a man quietly laments his life and hopes that one day things will come his way. Adding politeness to We are never coming back together results in Everybody knows, a song where a man lets a woman know he's breaking up with her in a very calm and poetic way. The change is even more apparent when the bitter Christmases when you were mine turns into the (slightly too) sweet memories of Christmas brought by Something about December.
Finally, and on the other side, White Horse works better with the anger in. While this song is about a woman enraged at a man who let her down, taking the meanness out results in the hopeless laments of Yesterday's Hymn.
So there you have it. I hope it's clear that these are completely accurate results, that everything I've done here is perfectly scientific, and that any kind of criticism from Ms. Swift's fans can be safely disregarded. But on a more serious note: I hope it's clear that this is only the tip of the iceberg, and that you can take the ideas I've presented here in many cool directions. Need a hand? Let me know!
Further reading
- Piotr Migdal has written a
popular
post about why the
King - man + woman = Queen
analogy works, including an interactive tool. - The base for my code was inspired by
this
tutorial on the use of
word2vec
. - The good fellows at FiveThirtyEight used this technique to analyze what Trump supporters look like, applying the technique to the news aggregator Reddit.